Lee Sedol vs AlphaGo
by Anders Kierulf
2016-02-02
Google’s AlphaGo beat the European Go champion Fan Hui in October, and Google has challenged Lee Sedol to a five-game match in March. How can we assess his chances?
Analysis of October games
As an amateur 3 dan, I can understand much of what’s going on in the five games AlphaGo played against Fan Hui, but professional analysis reveals subtleties and deeper issues:
- Myungwan Kim 9p: Review on AGA Youtube Channel (with a good synopsis on reddit).
- Younggil An 8p: Commented SGF of game 5 and video commentary of game 2.
Also, this PDF by the British Go Association includes some history of computer Go, more background on the match, and an analysis with comments by Hajin Lee 3p.
The conclusions I draw from all of this:
- Fan Hui made a number of mistakes that Lee Sedol is unlikely to make.
- While AlphaGo played very well, it did make some mistakes in those five games. Also, Fan Hui did win two unofficial games against AlphaGo (sadly unpublished).
- AlphaGo’s reading (looking ahead many moves to determine whether a plan will work or not) is very strong.
- AlphaGo sometimes mimics the play of professional players and follows standard patterns that may not be optimal in that specific situation. Professional players are more creative and will vary their play more based on subtle differences in other parts of the board.
- AlphaGo may not have a nuanced enough understanding of the value of sente (having the initiative).
- AlphaGo doesn’t show deep understanding of why a move is played, or the far-reaching effects of a move.
So I’m confident that the October version of AlphaGo was weaker than Lee Sedol. And those five games only give us a limited view of AlphaGo; there are probably more weaknesses to be discovered. Ko was only played once; AlphaGo did well, but we don’t know how it will do in a complex, protracted ko fight. We don’t know how it will do when the fighting gets more complex. We don’t know how it will do when the board is more fluid and multiple local positions are left unresolved.
March version of AlphaGo
Google has five months to improve AlphaGo. So what can they do?
During the Deep Blue match, engineers could make adjustments to Deep Blue’s algorithm between games, such as fixing a bug after game 1. For AlphaGo, it may not be as easy. For example, move 31 in game 2 (shown below) was a mistake by AlphaGo (see Myungwan Kim’s analysis). It’s the right local move under the right circumstances, but AlphaGo doesn’t have a sufficient understanding of that board position. How can they fix that issue? Feeding that position to the neural network won’t help balance out what it has learned in 30 million other positions. There’s no quick fix for that kind of mistake.
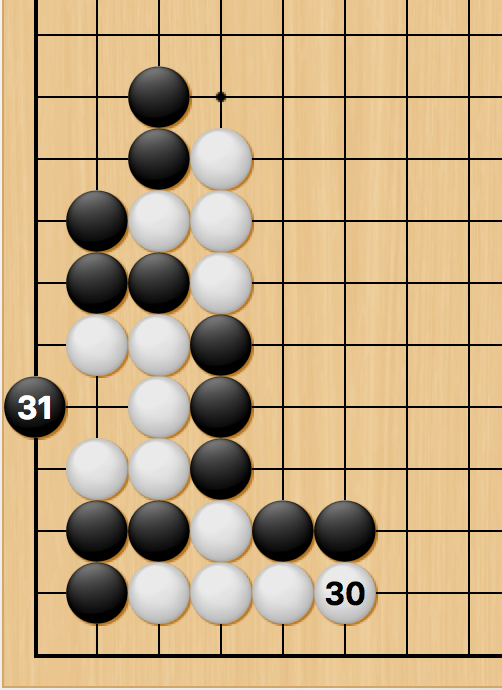
Avoiding specific mistakes is easier. AlphaGo was not using an opening library in October, but Google could easily add that by March, making it at least possible to adjust play between games so Lee Sedol won’t be able to exploit a particular joseki mistake in multiple games.
There are many other improvements Google can make before March:
- Google can refine AlphaGo’s neural networks. They used 30 million positions to train the value network for the Fan Hui match — they can use 100 million for the Lee Sedol match.
- They can add extra training for the rollout policy. And then feed the improved rollout policy into the training of the value network.
- They can fine-tune the balance between rollouts and the value network.
- They can throw more computing power at the match itself. And the match will likely have longer time limits, so AlphaGo can calculate more during the opponent’s time.
- And more. Google has a strong team that wants to win, and they’ll have other ideas up their sleeves.
Google has also expressed confidence, and they chose the opponent and timing for this next match. So I’d expect the March version of AlphaGo to be significantly stronger than the one that played last October. Its reading is going to be even better. Its assessment of the global position will be improved.
The main question is whether those improvements will be enough to remedy or at least balance out the weaknesses seen in the October version. Google made a huge leap forward with AlphaGo, creating a qualitative difference in computer play, not just a quantitative one. It’s hard to tell what another five months of work and neural network training can do.
Conclusion
The AlphaGo from last October was very strong, but probably not strong enough to beat Lee Sedol. With five months of work, I think AlphaGo is going to be a different beast in March, and it will be a very exciting match. If I had to bet? Lee Sedol will lose a game or two but win the match.